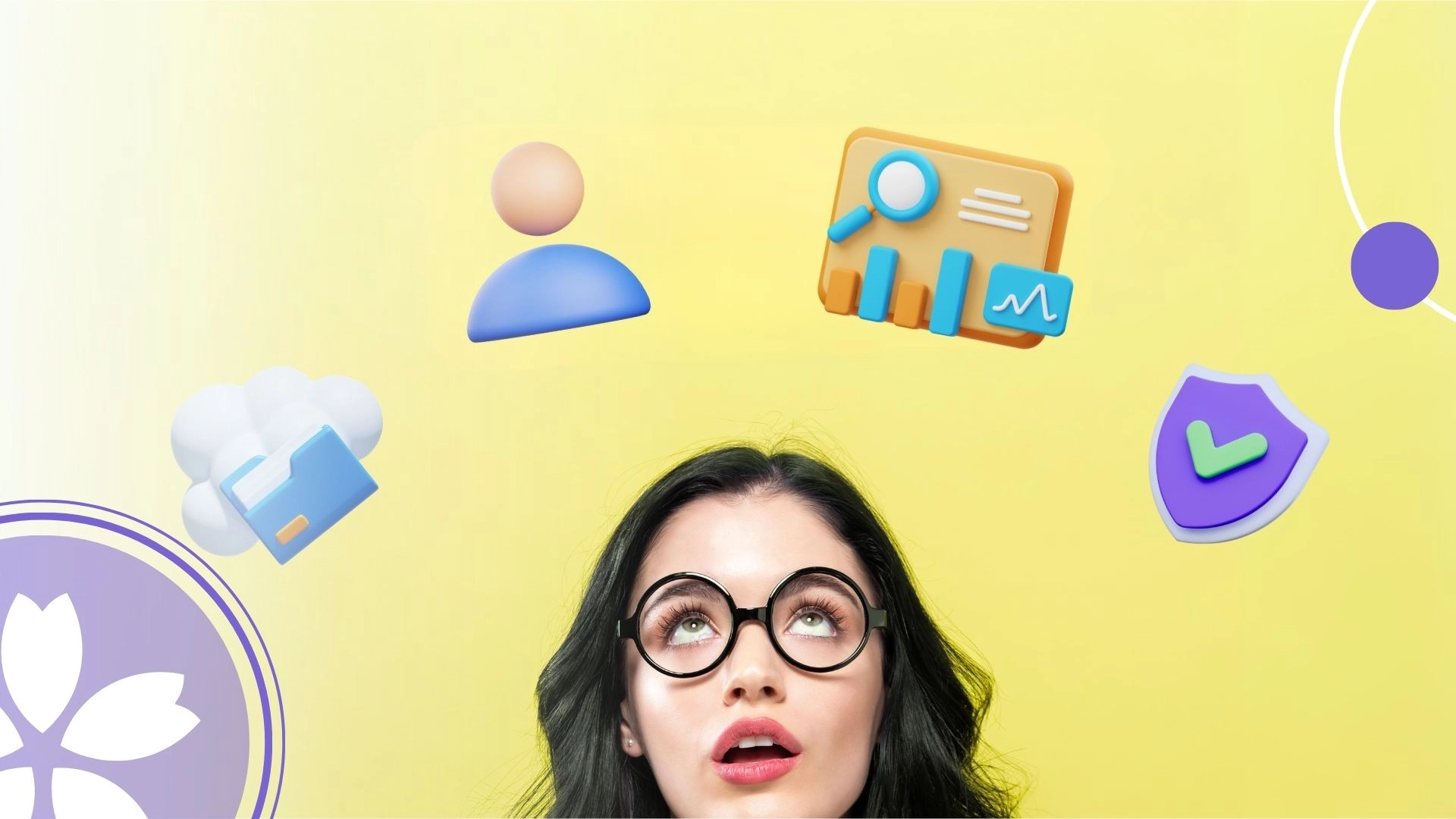
What to Look For in an Experimentation Platform (Beyond Features)
How to make a strategic decision and look for alignment, not just a laundry list of functionality
Table of contents
In a recent post, we covered a short list of questions to guide your build vs. buy decision for experimentation. Building an experimentation platform from scratch requires specialized expertise and a meaningful dedication to headcount for the task. Few organizations have these specialized resources - but the pace of innovation in commercially available tooling has removed any need to compromise on capabilities.
This post will focus on how to evaluate experimentation tools, assessing how well each vendor and solution fits your context, needs, and goals. By making evaluations based on these strategic considerations, you'll avoid buying a fancy list of features that will never be used because of a more fundamental misalignment.
Who Needs To Run Experiments?
Think about who in your organization should be able to set up and run tests autonomously. Is it product teams? Marketing professionals? This will translate into requirements around both UX and architecture.
There are two main pitfalls to avoid:
- A highly technical tool that lacks accessibility for business users.
- A shallow tool that demos as accessible, but poor integrations and lack of flexibility lead to limited adoption.
In my past experience as an experimentation consultant, I saw many data and product teams attempt building in-house tools, while their marketing team counterparts went out and bought a legacy A/B testing tool from a vendor. The marketing team would have some ability to get tests live on their own, but trust in the results would be scarce. The internal tool users, on the other hand, had reliable metrics direct from the source of truth but required a lot of manual work and analysis to get tests live (never mind the cost of building and maintaining the platform itself).
In one household-name FinTech company I advised, this even led to internal "statistics wars". Competing teams reported different measures of inference to executives, who were never sure how to interpret or compare p-values and Bayesian "probabilities to be best". A particularly divisive website redesign project eventually boiled over into two teams (Design and Marketing) hiring different consultants to evaluate their experiments and explain why revenue metrics were so unexpectedly down after launch.
Key takeaway: The most trusted experiment platform wins. Hold out for the right tool that can balance flexibility and accessibility and unify analysis efforts on a single platform.
![]() |
β
What Metrics Can It Measure?
Look at what data sources and metrics matter most to your business. Can the AB testing tool integrate with those systems and leverage the exact KPIs that influence strategy and reporting? Beware of tools that rely solely on client-side or clickstream data, as you'll want to track key business metrics like revenue, margins, or activation.
Since most businesses report on these most important metrics directly from a data warehouse, the gold standard for an experimentation platform should be a fully native integration with the data warehouse. When data needs to be egressed to the experimentation platform, room for discrepancy arises.
These discrepancies are almost inevitable when comparing source-of-truth measurements with clickstream approximations, e.g. revenue as reported in GA4 vs. an exact revenue number from a data warehouse. Chasing them can become a huge time waster, or worse - kill trust in experiment results entirely. Relying on data from GA4 for experiment analysis is an especially problematic example because of how Google Analytics samples data: with an algorithm called HyperLogLog++ that is essentially wholly incompatible with the statistics underlying experiments.
Because most legacy A/B testing tools are ill-suited to measuring metrics from a source of truth, some users decide to discard the analysis capabilities of their software entirely and try manually using external "statistical calculators" to derive more reliable resultsβa surefire way to introduce bottlenecks in analysis or potential errors from users without background knowledge of statistics.
![]() |
Is The Solution Scalable?
Consider your ambition for experimentation over the next 1-2 years. How widespread will testing be? How many tests will you want to run? The tool should be flexible enough to meet your future needs at scale.
Legacy A/B testing tools are often unable to scale because they implement tests on the client-side, using Javascript snippets, and mostly leverage WYSIWYG visual editors to build treatments (two topics I've written about extensively, if you'd like to click those links).
But even modern tools built on the data warehouse can have scalability issues. Analyzing experiments requires massive joins of data and "combinatoric explosions" - computationally intensive tasks that can quickly become expensive without extensive optimization. This is why an important part of our "pitch" at Eppo is our commitment to data warehouse-native architecture: it is the only thing we do. For tools where this approach is an afterthought or tack-on, the hidden costs of ownership can be an unwelcome surprise as you scale.
Vendor Support and Expertise
Lack of statistical knowledge can be a core cause of failure-to-launch for cultures of experimentation. Even within the data science space, experimentation and causal inference is too often a "niche" topic, with relatively few specialists on the talent market. As a result, your vendor should be a valuable contributor in the effort to educate your teams.
Assess their degree of in-the-field experimentation experience and included support. Will they be in your Slack instance with you helping navigate questions and concerns? Will they be able to show true know-how (not just product knowledge) and win over skeptical audiences when explaining nuanced topics like statistical power, experiment design, or interaction effects?
It may also be relevant to look for partners who have experience leading experimentation in similar environments. Tools that are entirely informed by experience at a FAANG-level tech company may be impressive, but translate less well to the teams and needs of an earlier-stage startup. (This is part of why we're proud of our diversity of experience at Eppo - we're informed equally by big companies like Airbnb and LinkedIn and startups like Storyblocks, Angi, or Big Fish Games)
Investigation Capabilities
Briefly dipping into the feature list to look at one underappreciated area: beyond basic reporting, can the tool provide drill-down insights into segments and slices? How easy is it to diagnose the impact across metrics? Strong experimentation requires strong analytics.
Experimentation inevitably delivers a lot of surprising learnings too. If we knew every outcome that might be interesting before running the test, it wouldn't be much of an experiment. Watch out for platforms that require segments and slices to be pre-defined before running an experiment since the lack of flexibility will limit that learning and exploration.
![]() |
β
Security and Privacy
Finally, check for alignment early on the security and privacy, since these are the #1 issues that will stop a new tool evaluation dead in its tracks, no matter how much the end user team likes it. Review each vendor's standards, policies, and capabilities around data privacy, including GDPR compliance. Do they align with your own governance?
Parting Thoughts
Ultimately, how will you position experimentation (and this investment) to your executives? Is experimentation a way to express impact? A way to derisk your engineering investments? A way to find extra money in the couch cushions? A way to learn quickly?
Features are important, but they're a surface-level part of the decision. Features alone will almost inevitably fail to capture the true make-or-break considerations in buying something as technically and culturally nuanced as an experimentation platform. Think of this as a strategic decision based on alignment between your organization and a potential vendor - not just a "job to be done".
With a clear understanding of your internal use cases, data infrastructure, and business priorities, assessing experimentation vendors against criteria like these will ensure you select the right solution to meet both your immediate goals and scale-up vision.