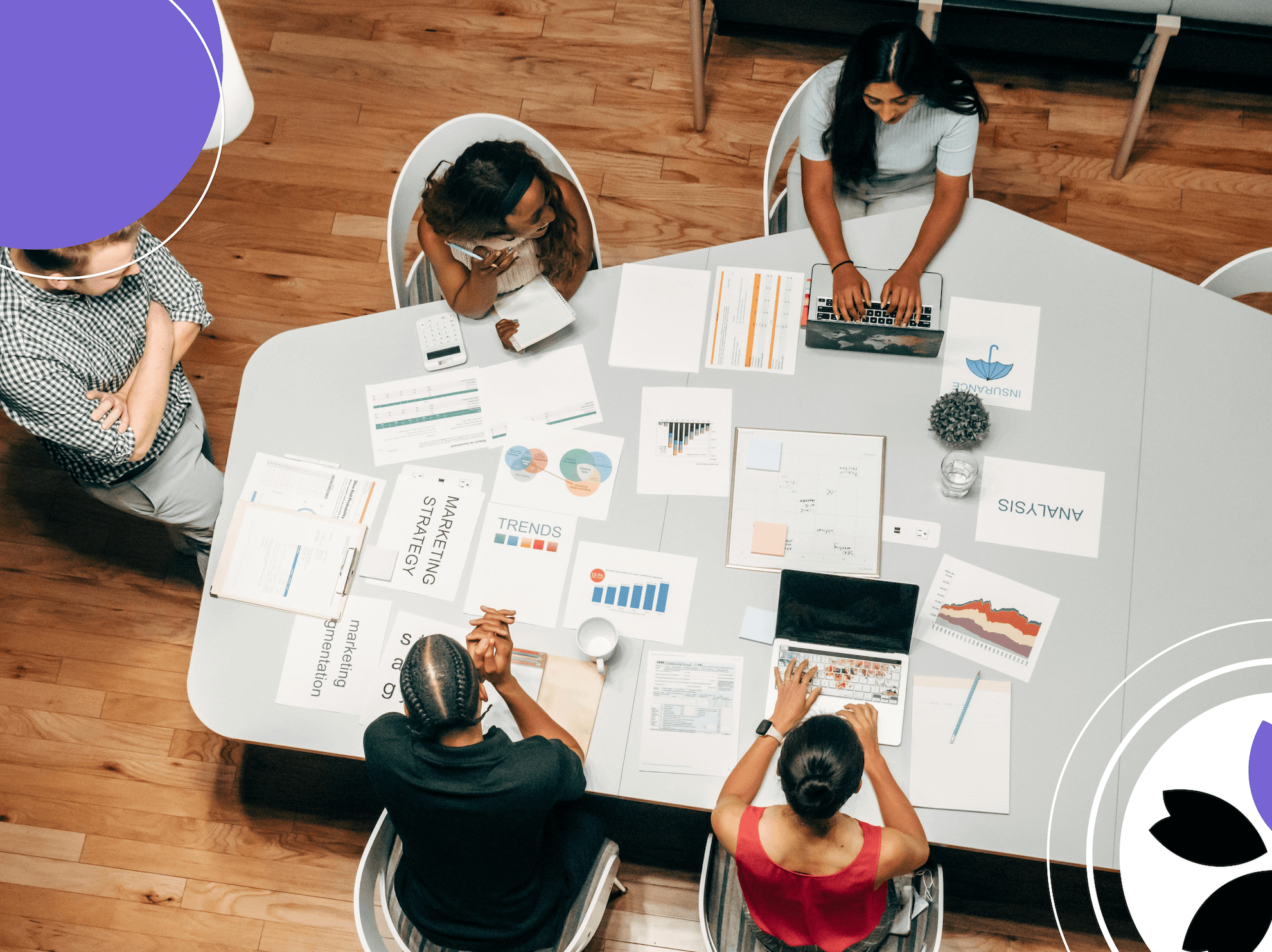
What is A/B/n Testing? Easy Guide with Examples
Unlock data-driven growth. Understand A/B/n testing, its benefits, and how to implement it effectively. Get practical examples and expert tips.
Table of contents
In today’s highly competitive SaaS landscape, guesswork simply won’t cut it.
A/B/n testing has emerged as a key experimentation strategy that’s helping tech leaders like Google and Microsoft dominate the market.
If you're frustrated with lackluster results and stagnant conversion rates, consider this: The root cause may be an overreliance on gut feelings and outdated assumptions.
In today’s article, we’ll learn all A/B/n testing and how it can actually have an impact on important business metrics like revenue and retention.
Here’s what we’ll cover:
- What is an A/B/n test?
- A quick 5-step guide to A/B/n testing
- Examples of A/B/n in practice
- Key benefits of running A/B/n tests
- Challenges of using A/B/n testing
- Best practices for effective A/B/n testing
Let’s get started.
What is an A/B/n test?
A/B/n testing is a way to compare multiple different versions of something (like a webpage, app feature, or marketing asset) and see which variation performs better. You create different variations by changing elements. Then, users are randomly shown one of these variations, and their behavior is tracked.
The version that produces the most desired actions (like clicks, sign-ups, or purchases) is the winner.
A/B/n testing builds on the classic A/B test (where only two versions go head-to-head). The "n" simply means you can include any number of variations beyond two. It's great for situations where you want to experiment with a wider range of ideas to find the optimal design for your website.
Implementing A/B/n testing: Step-by-step guide
A/B/n testing offers a structured way to discover what truly works best for your visitors. Let's break down the process into five easy steps:
- Step 1 — Hypothesis formulation: Define your goal and the specific metric you'll use to measure success.
- Step 2 — Test design: Start with your original format as the "control" and create additional variations, each with one key change. Determine the necessary sample size for reliable results according to your specific context (e.g. metric baselines).
- Step 3 — Execution: Use A/B/n testing tools to split your audience and gather data. Allow the test to run long enough to collect your determined sample size and hopefully observe meaningful patterns.
- Step 4 — Analysis: Analyze results with an eye to statistical significance — this will tell us how “surprised” we should be by the results and suggest if our results are “signal”, or likely just “noise”.
- Step 5 — Action: Implement the winning variation and take note of lessons learned about audience preferences, even from variations that didn't win outright.
Examples of A/B/n testing in action
Let’s take a look at some simple examples of how A/B/n testing can be put into action across various scenarios:
Example 1: Website design
Imagine launching a new product. You design several landing pages with different layouts, headlines, and visuals.
A/B/n testing lets you release all the variations simultaneously. Data reveals which page drives the most sign-ups or purchases. This eliminates guesswork and leads to a design optimized for conversion from the get-go.
Example 2: Email marketing
Subject lines can make or break an email campaign. A/B/n testing can help. Experiment with different variations in tone (formal vs. friendly), personalization (using the recipient's name), or CTAs within the subject line. Analyze open rates to see which version leads to more clicks.
Example 3: Product features
When creating a new project management tool, suppose you're considering three methods for users to mark tasks as complete: A checkbox (Variation A), a progress bar slider (Variation B), and a "mark as done" button with an animation (Variation C). A/B/n testing shines here, letting you compare these options directly.
You'll track metrics like task completion rate and average task completion time. For instance, Variation A might lead to the quickest task marking due to its simplicity. Variation B could encourage more thorough task completion with its visual progress indicator, potentially increasing the task completion rate. Variation C might boost user satisfaction by making task completion feel rewarding, reflected in positive user feedback.
After exposing different user groups to each variation, you analyze the data. If Variation B shows a higher completion rate and comparable completion time to Variation A, plus favorable feedback, it might be the best choice. However, if the efficiency of Variation A or the user satisfaction with Variation C outweighs B's benefits, they could be preferable.
The decision hinges on balancing efficiency, effectiveness, and user experience, aiming for a feature that aligns with your tool’s overall goals.
Benefits of A/B/n testing
A/B/n testing enables you to go beyond hunches, making choices backed by hard data. Here's how it can transform your decision-making:
- Data-driven decisions: No more guesswork. A/B/n testing reveals which versions resonate best with your audience. You can confidently choose the winning design, message, or feature, armed with concrete results.
- Boosting engagement and conversions: A/B/n testing lets you experiment with every aspect impacting the user experience. By testing different CTAs, design elements, or even the tone of your message, you can uncover what leads to more revenue and retention.
- Fine-tuning with feedback: Think of A/B/n testing as a conversation with your audience. By tracking their behavior, you gain invaluable insights into what they respond to best. This allows you to refine your offerings over time, delivering experiences that truly connect. Unlike actual user interviews or focus groups, you’ll see their behavior in the real world - not biased feedback that may or may not prove true after the interview.
- Reducing risk: Rolling out major changes without testing can be risky. A/B/n testing lets you validate ideas on a smaller scale before committing to a full-blown launch. This helps guarantee that the changes you implement will have the desired positive impact.
Challenges and considerations in A/B/n testing
While useful, A/B/n testing comes with potential hurdles to be aware of. Here are some key aspects to consider:
- Complexity of analysis: When dealing with multiple variations, it's crucial to make sure you plan for sufficient sample sizes to determine if the results reflect genuine trends or just chance fluctuations. The more variations you test, the larger of a total sample you’ll need (and the longer you’ll need to run the test).
- Managing more variables: Testing multiple versions means juggling more elements. It's important to maintain careful control of your test conditions — changing too many options at once can make it difficult to isolate what's driving the results.
- Avoiding pitfalls: Factors like sample size limitations or accidental overlap when running multiple tests simultaneously can muddy the waters. Ensuring a well-structured testing plan and recognizing common errors is essential for reliable insights.
- Sample pollution: Your results can be skewed if users are exposed to multiple variations simultaneously (for example, if they revisit your website or encounter your campaign on different platforms). Advanced experimentation tools like Eppo will solve for this.
- The importance of tools: A good A/B/n testing tool is invaluable. It manages traffic distribution, data collection, and analysis, making sure that your tests run smoothly and the results are easy to interpret.
Best practices for effective A/B/n testing
Get the most out of your A/B/n testing efforts by following these guidelines:
- Focus on impactful changes: Start with a clear hypothesis and test variations that could make a meaningful difference. It's better to change one significant element at a time rather than tweaking multiple minor aspects.
- Statistical significance is key: Don't just go by raw numbers. Look for statistically significant differences in your results. This helps validate that your winning variation is truly better, not just a likely result of random chance.
- Adequate sample and duration: Your sample needs to be large enough to reflect your audience accurately. Use online calculators to determine the appropriate sample size. Also, give your test enough time to gather meaningful data and let patterns emerge.
- Test, learn, repeat: A single A/B/n test is just the start. View testing as a continuous process. The insights gained from each test can inform and refine your next iteration, leading to ever-improving results over time.
- Don't forget context: Remember that user preferences can shift based on seasonal trends, current events, and a multitude of other factors. Adapt your testing strategy to account for how your audience's needs may change.
Next steps
You know that A/B/n testing unlocks valuable insights for your business. But turning that knowledge into action can feel complex.
Eppo makes it a seamless process, empowering you to run reliable A/B/n experiments without the need for extensive data science expertise.
Data you can trust
Traditional tools may leave you questioning results. Eppo prioritizes statistical rigor, so you can make confident decisions. Our end-to-end platform and warehouse-native architecture deliver clarity and transparency, driving actionable insights that impact your bottom line.
Getting started with Eppo is easy:
Once you’ve tested out Eppo through a quick demo and decided to start using Eppo in your own system, our warehouse-native platform will integrate with your own data warehouses (Databrick, Redshift, BigQuery, Snowflake, and more).
Next, you’ll be able to use Eppo’s SDKs to deliver A/B/n tests to your users and start experimenting immediately.
After setup is done, you can start making use of Eppo’s features:
- Focused planning: Define clear objectives and craft insightful hypotheses. Eppo's sample size planning tools and metrics alignment ensure each test drives meaningful results that directly impact your business goals.
- Flexible experiment design: Elevate your testing capabilities with Eppo's robust feature flagging and sophisticated design options. Tailor your experiments with precision, utilizing various testing environments, allocation strategies, mutually exclusive layers, and holdouts.
- Confident launches: Eppo's integrated diagnostics and real-time monitoring pinpoint potential issues early, safeguarding the integrity of your experiments and maximizing your investment.
- Empowering analysis: Eppo's powerful statistical engine delivers reliable and easy-to-interpret results, transforming raw data into actionable recommendations. Delve deeper with flexible exploration tools to uncover even more granular insights.
Ready to start running A/B/n tests? Book a Demo and Explore Eppo.