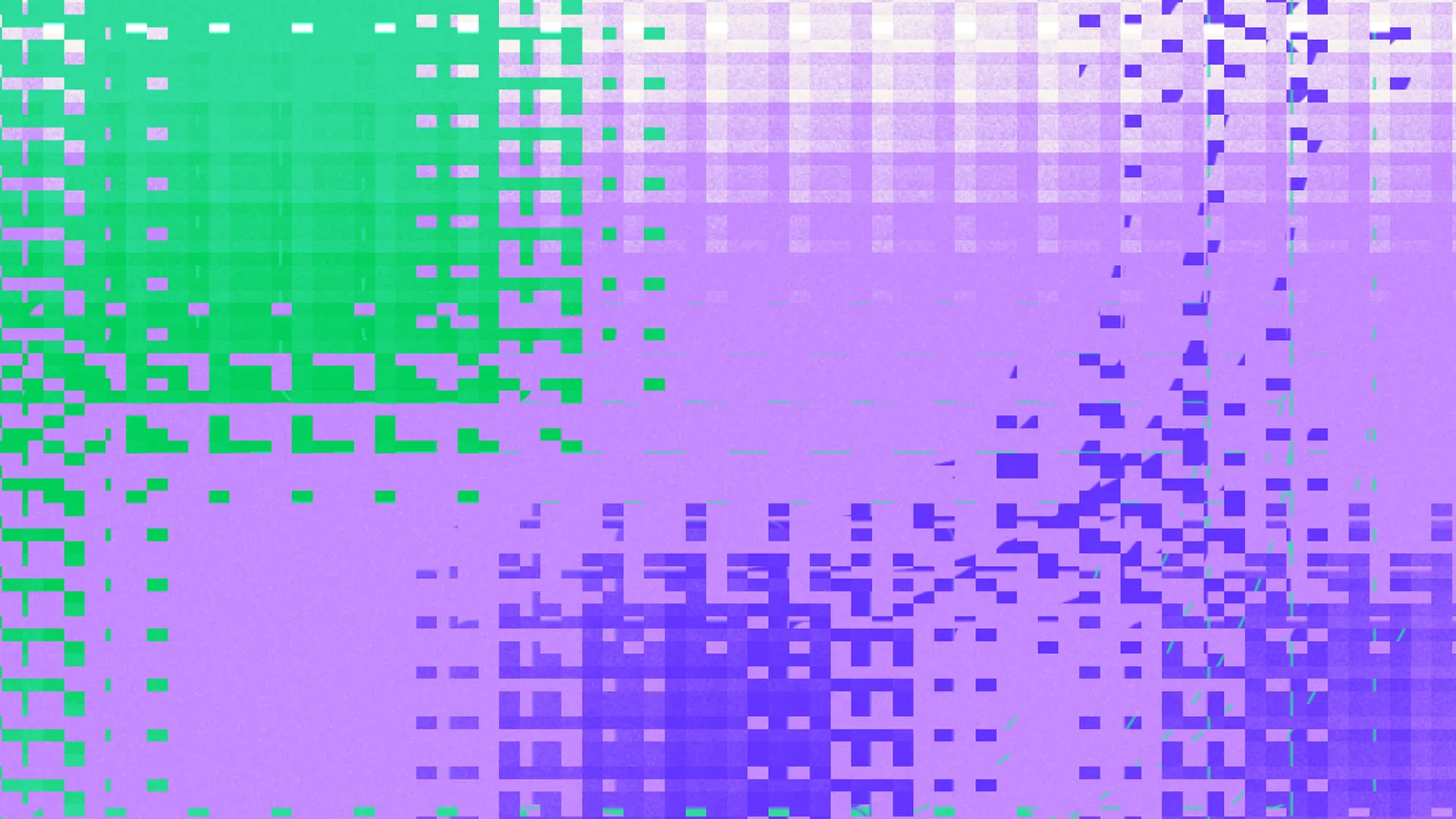
AI/ML
How Netflix, Lyft, and Yahoo use Contextual Bandits for Personalization
Inspiration from some of the world's biggest tech orgs
Learn more
As part of Eppo’s Humans of Experimentation conversation series, we sat down with Shailvi Wakhlu, an accomplished data leader, international keynote speaker, and angel investor.
In past roles, Shailvi was the Head of Data at Strava, where she ran a 27-person product analytics and machine learning team; and the Head of Analytics at Komodo Health, where she managed a team of data scientists. Shailvi has spent her entire career building subscription products for both enterprise and consumer companies.
She is currently teaching a course on Data Storytelling for CoRise.
In this conversation, Shailvi explains:
Why data storytelling is key to the success of a data practitioner
Why machine learning requires experimentation to show quantifiable business benefits
How experimentation can help generative AI move into “the real world”
I feel like our industry is not very streamlined in how different companies think about this question, which is unfortunate!
There’s a lot of confusion. Even when people are applying to data roles, they're not exactly sure where the team sits. Where a data team sits can affect the outcomes it can produce. Inherently, data teams are responsible for data, but how a company splits up the responsibilities amongst different teams and different functions varies quite a bit.
In my case, the last four companies I worked at were all companies where data was the product. Strava, Komodo, Salesforce, Fitbit were all companies that are very data-rich, and they wouldn't have a business if they weren't sitting on massive amounts of data.
So the data teams in some cases were merged with core product and technology teams, and the responsibilities adapted accordingly. I personally love models where data work happens in a centralized data org, so the data engineering, governance, all the infrastructure pieces, analytics, machine learning, visualization - all reside in one place. It aligns accountability and resources, and it makes data teams much more efficient.
Many people in the data field come from technical backgrounds, and data storytelling is not really set up as a skill that people practice. It’s considered an add-on, at best.
I personally feel it's incredibly important for the success of a data practitioner to be great at data storytelling. I benefited a lot in my career from the fact that I was good at it and comfortable with it. I understood the components of what data story can make big impact, and get the right buy-in. Data storytelling is definitely an art, but most parts of it can be broken down into a science.
How do you prioritize the specific needs of your audience? How do you adapt your communication methodology? It should be based on a deep understanding of the people, the problems, and the data involved. I believe that effective data storytelling customizes these pieces, making them relevant to a specific audience, so they can actually understand what matters and make decisions appropriately.
I still remember the awe and excitement that I felt launching some of those experiments.
When I was an IC, data storytelling was more about presentations and compelling visualizations. But as a data leader, in addition to those pieces, it's about setting the tone for a data culture, and educating the right stakeholders so that you and the business have everything needed to actually make good decisions.
Ultimately it is about getting the business what it needs, through the support of data. So people have to have that basic level of understanding of what the data story is actually telling them. They have to feel invested in it. They have to feel like they can trust it. And that's what brings them to actually act on it.
Some of my most memorable experiments were actually when I transitioned from software engineering to analytics for the first time and ran my first experiments.
At Monster.com, analytics was a new function at my location. Experimentation was a new muscle. Some of the early experiments that I ran were about tweaking the algorithm that was used to send job-seekers more relevant alerts and try to get them back on that platform. We came up with a good hypothesis that people are still likely to find relevancy in jobs that are located in their closest big city, and that we’d find more matches for them in the big city rather than in their current small town. We made this change in our job alert algorithm, and did an A/B test to see if this worked. We noticed a significant lift in our primary metrics (enough that it temporarily broke some of our systems due to the increased volumes!), while maintaining our thresholds with counter metrics. This was a big win and resulted in many further experiments that helped improve our algorithms.
I still remember the awe and excitement that I felt launching some of those experiments. I remember that feeling of being able to see the impact on things that we as a company had not yet made a massive effort to try to optimize.
I'm so grateful that I began my career in analytics with a really large user base where you could run very effective experiments, and didn't have to wait weeks to get a result. You could know within a couple of days whether a feature change was successful or not.
Some of the biggest problems faced by companies are at the data strategy level. Companies shouldn’t drag their heels on coming up with a clear strategy of how they will use, maintain, and store data.
If you have a really large user base, and your company is dependent on data, you need to be very intentional! You can’t just shove data teams around, and shove around responsibilities - that’s a recipe for disaster. You can't make inconsistent decisions on how to invest in engineering tooling and all the pieces that are required for scale.
Fear of failure should never stop you from experimenting. If you have a good hypothesis, you should experiment.
You need to align the incentives of product teams so that they see the value in being data-informed, and not just rely on their gut. Because then you run into situations where you are years into making bad decisions, and a simple experiment can highlight that you've been making big mistakes the whole time.
If you ran that experiment years ago, you would have avoided making 10 other decisions that were aligned with that incorrect assumption.
I have worked at companies where experimentation was not the norm, and people were almost scared of it. They were wondering, will I have to experiment on every single change that I make on our platform?
For me, it starts with getting clarity: Are you clear on your goals? What are you trying to optimize? What will be useful to your users?
Once you answer that, you’ll have a better hypothesis around which changes to explore. Then you can set up experiments that will give you a definitive answer about whether you’re improving your product, and by what quantifiable metrics.
Fear of failure should never stop you from experimenting. If you have a good hypothesis, you should experiment. You should experiment because you want to learn the truth about what your customer cares about. That way, it's not just reliant on conventional wisdom, which may be just based on intuition, or based on what users cared about three years ago when you launched the company.
In my last role, by the time I left, we had reached that stage where we were almost running too many experiments. Experiments are expensive to run, and they have an overhead cost associated with them.
So you have to come up with some guidelines for when not to run an experiment. If the cost is too high, the signal is expected to be too weak, or if you have no prompt to believe a tiny change will result in big benefits, you shouldn’t bother running an experiment. A change that requires 8 months of testing to get stat-sig volumes should not be part of an A/B test. Fixing a typo does not require an A/B test.
When I worked at Salesforce, they didn’t have an experimentation culture. In some ways, that is common for B2B businesses, because they often have the mindset of: let’s build what the customer asks us to build. This makes sense sometimes because their individual customers are businesses that sign large dollar amounts of contracts.
Consumer companies can’t do that. If you ask millions of customers to tell you what you should build, you're going to get a million different answers. So you have to make decisions based on actual product usage. And that's where experimentation is incredibly useful because it is often easier to show the user what they might want, and measure their reaction, than ask them and try to parse their answer into a product feature.
So with Salesforce, or at least the business lines that I supported, I was able to convince them of the value of experimentation by pitching the “show vs ask” mindset. These specific business lines were a B2B2C play, and the number of end users who experienced our product was really high. Additionally, our paying customers (enterprises) couldn’t tell us what their customers (individuals) wanted with 100% certainty either. So experimentation based on UX research was the way to go!
I also made the ROI pitch that small optimizations were going to go a long way for our customers, who we serve through their customers. So there was that sort of equation mapping, where we showed what a 1% change would look like in terms of our ability to retain our customers, grow our customers, and grow our seat sizes.
Some of the lowest-hanging fruit, especially for consumer companies, is making simple product optimizations. And again, that depends on how good your understanding is of your users and what they actually care about.
So, If you are trying to create more dynamic content for them, or trying to customize notifications for them, you’ll need to run experiments.
The more you use machine learning to figure out what someone should see based on who they are, you also have to experiment to quantify the improvements you can make to certain metrics.
Do you care about getting more subscribers? Do you care about people spending more time on the platform? Machine learning teams are heavily incentivized to quantify, through experimentation, their ability to positively affect key metrics, especially since so much of their work starts off within the innovation bucket. As we are seeing with generative AI right now, many ML models are built to explore various possibilities and build capabilities that take advantage of new techniques early. But ultimately, the work has to have quantifiable business benefits or ML teams won’t be able to attract the right investment.
You need experimentation to quantify the positive impact that you see through those ML changes for the core metrics that you care about.
There are going to be a lot more companies that use generative AI as a part of their product.
Customer support could be a very good use case. It has always had some components that were at least somewhat automated. We’ve all chatted with support bots before. But now, when you combine that use case with really large language models that can process all the knowledge from past customer support cases that have been solved, there is a greater likelihood of more relevant and accurate solutions. AI has the capacity to generate much more helpful responses than old-school bots.
But you have to experiment to optimize and quantify how that is playing out. For example, how many times do people still spin their wheels because they are being given incorrect information? ChatGPT can sound really confident when it's telling you something wrong!
You need to quantify the ways that the AI is saving you time and money, where the guardrail metrics are taken care of, and the accuracy of what is being communicated is not negatively impacted.
If you don't measure it, you could end up making a lot of mistakes. This is all still new. We are still figuring out what the most effective use cases are. What's the MVP of something that we can implement tomorrow and actually get value from? That is still something under review.
There are plenty of interesting use cases that truly help people. But you have to experiment and see how those are playing out in the real world.
100%. But in a lot of traditional experimentation, you have one fixed thing that you're comparing with another fixed thing.
With generative AI, the “fixed thing” is pretty large. It could be a service and harder to measure if you pick the wrong metrics. You could be comparing one service to another service, like in the customer support example. So the experiment setup gets a little more complicated, where you have to make sure you’re measuring the right thing. You can’t measure a set of tiny details, because generative AI creates something slightly different every time - so you have to measure the fundamentals of what the service is providing.
I think it's good that there's so much attention on AI right now, because it's drawing more investment in upstream problems. People have started caring more about how data gets generated in the first place. They’ve started caring about pipelines and infrastructure and data quality.
I'm thrilled to see our industry investing in that! It’s shifted in a positive direction where people don't just care about the pretty dashboard, they care about everything that has to happen correctly before you get to the pretty dashboard, so you can actually trust what it's telling you.
And with AI, the stakes are even higher, because you risk training it with junk. You're going to get junk if your pipeline is broken, or if your governance is flawed. You’re then not going to use AI to its greatest potential, and that would be a real shame!
Click here to sign up for Shailvi's course on Data Storytelling at CoRise.