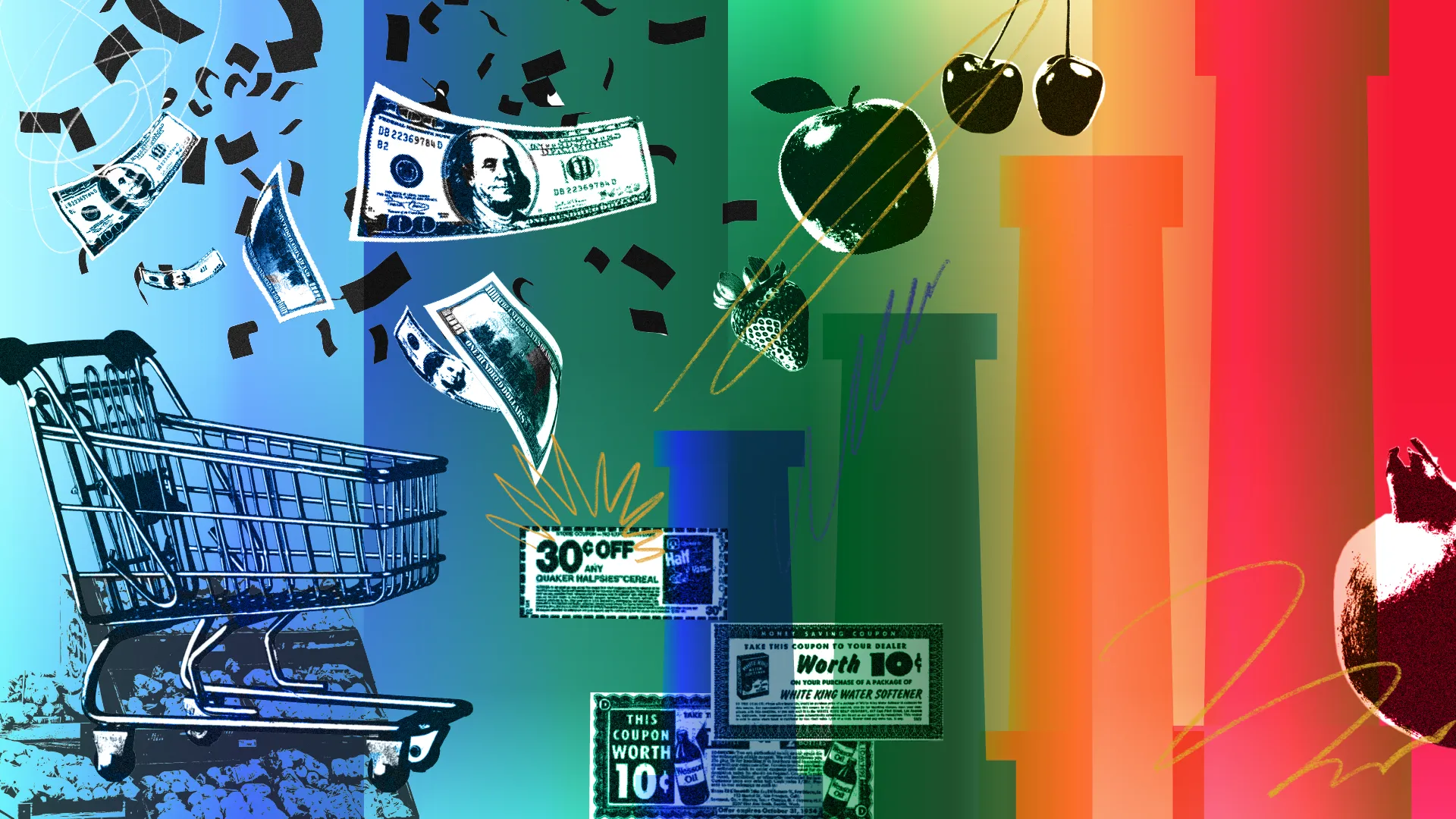
A/B Testing
Optimizing for Real Business Impact: A Strategic Framework for Ecommerce Experimentation
Learn more
If you’ve run A/B tests, you’re probably familiar with using sample size calculations to plan how many participants you need and, thus, how long you should run your test.
Sample size calculations are essential (and the first major point I’ll cover), but the biggest mistake most teams make is stopping there.
In fact, of all the A/B test planning guidance I’ve given teams (many teams!), it’s what to do next.
To plan proper, high-signal experiments, you must consider a few other factors about your customers.
They’re not complicated, but if you follow this advice, you’ll drastically improve the quality of your experiments, your ability to learn from your customers, and the pace of high-quality innovation.
And even if you have a unique situation not covered here, you’ll learn a lot about how to plan your own unique experiments.
Unfortunately, most teams experimenting out there do not have the in-house knowledge needed to help them figure out their own situation.
The customer features we will explore today are what I call “Runtime Modifiers.” Each exists on a spectrum that moves your required runtime up or down.
By adjusting your experiment runtime based on these customer features, you will:
Let’s dive into each feature that might define the types of customers you’re working with.
Most people know about the number of relevant customers you have to experiment with, which is called your sample size. Consider two extreme examples.
Sidebar: “Relevant” is an important addition here because you should only learn from customers who might actually be impacted by your changes (more irrelevant customers just add noise).
Small samples
Small samples tend to be noisy. It’s just luck of the draw, and chances are, you’re going to get a lot of wildly different customers in a small group. Get your hands on any power calculator like this one from Booking.com, and you can check it.
Here, I checked the impact I could detect with 100 customers (on a 10% baseline). It’d take a whopping 191% lift for me to detect that signal!
Large samples
Getting more customers means better representing what most customers tend to do or what’s “average.”
Using the same power calculator settings, bumping my customer sample to 1 million means I can now detect a 1.5% uplift. That’s a 127x reduction in the signal size we can detect.
So, generally speaking, the more relevant customers you’ve got, the less time you’ll need to run your experiment.
I’ve been a bit cheeky and ignored an assumption in the section above.
It’s not just the total number of customers you can get; you don’t just start your experiment and magically get that sample.
Participants enroll slowly over time. You need to wait! How long depends on how they enroll over time, and I’ve regularly encountered two specific patterns.
Pattern 1: Regular enrolment over time
The enrollment pattern that most people think of when they run A/B tests is “regular enrolment over time.” This pattern refers to a situation where you get the same number of participants enrolling regularly. For example, having 1,000 new participants enroll every week.
If you were to look at the daily count of customers entering your experiment, you tend to see something like this:
There may be weekly highs and lows, but they tend to be regular, so about the same number of enrollments show up each week.
I’ve encountered this pattern mostly in B2C scenarios, such as at Canva or Meta, where new users and potential customers show up regularly over time. It’s definitely common in B2C situations, such as when shoppers are on an e-commerce site.
If you re-read section 1 about sample size, you’ll see it assumes this pattern.
Pattern 2: Skewed enrolment over time
This pattern is defined by customers mostly entering early on (with a long tail after that). For example, 90% of your customers might enter the experiment in the first few days and the rest over the next few weeks.
If you were to look at the daily count of customers entering your experiment, you tend to see something like this:
I first encountered this pattern when working on the supply side of Booking.com’s business, building the products and services that helped accommodation providers (like hotels) upload rooms to be bookable to potential customers on the demand side.
I’ve also seen it several times since then and definitely see it more often for B2B products providing admin or management software.
Before we jump to the other considerations below (wink wink), if you pair these patterns only with the sample size, you will see that you can typically run experiments with Pattern 2 much faster than Pattern 1.
Why? Say you want about 100 customers in your experiment. With Pattern 1 (regular), if ten new customers show up each day, it will take you a week to get to 70%. With Pattern 2 (Skew), you might see 70% of your customers show up on Day 1!
So, in general (but wait for more!), you can run shorter experiments when your customers mostly show up at the start.
However, there’s a common contrasting feature to the patterns by which customers enroll in your experiment: customer frequency-of-use. Let’s look at two extremes.
High frequency-of-use
Customers entering experiments in a skewed way (e.g., 70% on day 1) tend to be high-frequency-of-use customers. That is, they use the product a lot. Like, every day. Using the example I shared from Booking.com, hotel staff tend to be updating their supply almost every day! Similarly, think about how you use your work software, such as through emails.
The tricky thing with high-frequency-of-use customers is that they are hyper-sensitive to change. They use the product so much that many interactions become instinct-based (like learning to drive a car). So, when change is introduced, you get something called a ‘novelty effect.’
Novelty effects can be positive, such as a new shiny button that everyone wants to click, or negative, like moving a button so no one can find it anymore. Either way, novelty effects are responses that suddenly spike when customers get something new and then fade as they get used to the change.
High-frequency-of-use customers tend to be significantly more susceptible to producing novelty effects.
So, in general, if you have high-frequency-of-use customers, you will need to run your experiment for longer (compared to low-frequency-of-use customers) to allow for novelty effects to pass.
Low frequency-of-use
Conversely, when you’ve got participants enrolling at a regular rate (e.g., 100 new customers per day), you tend to be dealing with low-frequency-of-use customers. Customers use the product relatively infrequently, such as once per month or year. Think about websites you’d use to book a holiday.
The nice thing about low-frequency-of-use customers is that each time they visit your product, it’s like a new experience again. They’ve probably forgotten a few things and are expecting to do some thinking and make some mistakes.
Low-requency-of-use customers tend to be slightly more oblivious to the changes you’ve made in an experiment.
The final customer feature I always check when thinking about runtime is the time it takes for them to trigger the value you expect and for it to show up in your metrics (usually your primary metric).
Whatever your business, there are actions customers take that are clear signals of value. Typically, it’s a point of purchase. Depending on your business, however, the path to get there can be quite fast or slow. Consider each.
Fast time-to-trigger
Fast time-to-value customers go from entry in an experiment to a potential value-creation action very quickly—I’d say in the range of minutes to days. Think, for example, of shoppers in a supermarket, buying something from Amazon, creating a design in Canva, or purchasing a plane ticket.
Slow time-to-value
Slow time-to-value customers, however, might have to wait a while before that value is realized, say weeks to months or even longer. For example, the Netflix team has to wait an entire month to see which customers continue or cancel their subscriptions. Or the time it could take a new Etsy seller to sign up to make their first sale.
You can probably imagine that having slow time-to-value customers typically means you have to run experiments longer. Why? You need to give your customers adequate time to get through their potential value cycle to understand if your changes have had a meaningful impact.
So, in general, when your customers have a fast time-to-value, you can run shorter experiments.
Now that we know how to consider these other factors, let’s test them with a couple of real-life examples.
Let’s assume for all cases that a power calculation using the primary metric and desired minimum detectable effect tells us we need a sample size of 500K to work with.
OK, let’s dig in…
Example 1: Testing a new payment UI on an e-commerce site (like Amazon)
In situations like this, relevant customers tend to:
So, we build up our runtime according to these like so:
Based on this, I’d suggest running the experiment for two weeks.
Example 2: Testing a new sign-up flow in a freemium business (like Canva)
In situations like this, relevant customers tend to:
So, we build up our runtime according to these like so:
Based on this, I’d suggest we run the experiment for at least four weeks.
Why? This choice comes from two weeks to get the desired sample and another two weeks to give them sufficient time to potentially make a purchase.
Example 3: Testing a new home-page UI in an enterprise software (like Microsoft Outlook)
In situations like this, relevant customers tend to:
So, we build up our runtime according to these like so:
Based on this, I’d suggest we run the experiment for at least one week.
Why? We get the desired sample in a matter of days. However, novelty effects could take a few days to dissipate. Combined, we’re looking at at least 5-6 days, at which point we could round up to account for any stragglers and as a safety precaution (e.g., to account for any weekly seasonality).
Remember, learning quickly and effectively from your customers will require tailoring your experiment runtimes to their unique features. Be sure to consider these four “runtime modifiers” in your planning.
Doing so will help you run higher-quality experiments and get you innovating and delivering value at a much faster rate!
I hope you’ll think them through next time you need to plan an experiment.
If you’d like some help, let’s connect and chat on LinkedIn or contact me through hypergrowthdata.com.
Until next time, thanks for reading! 👋