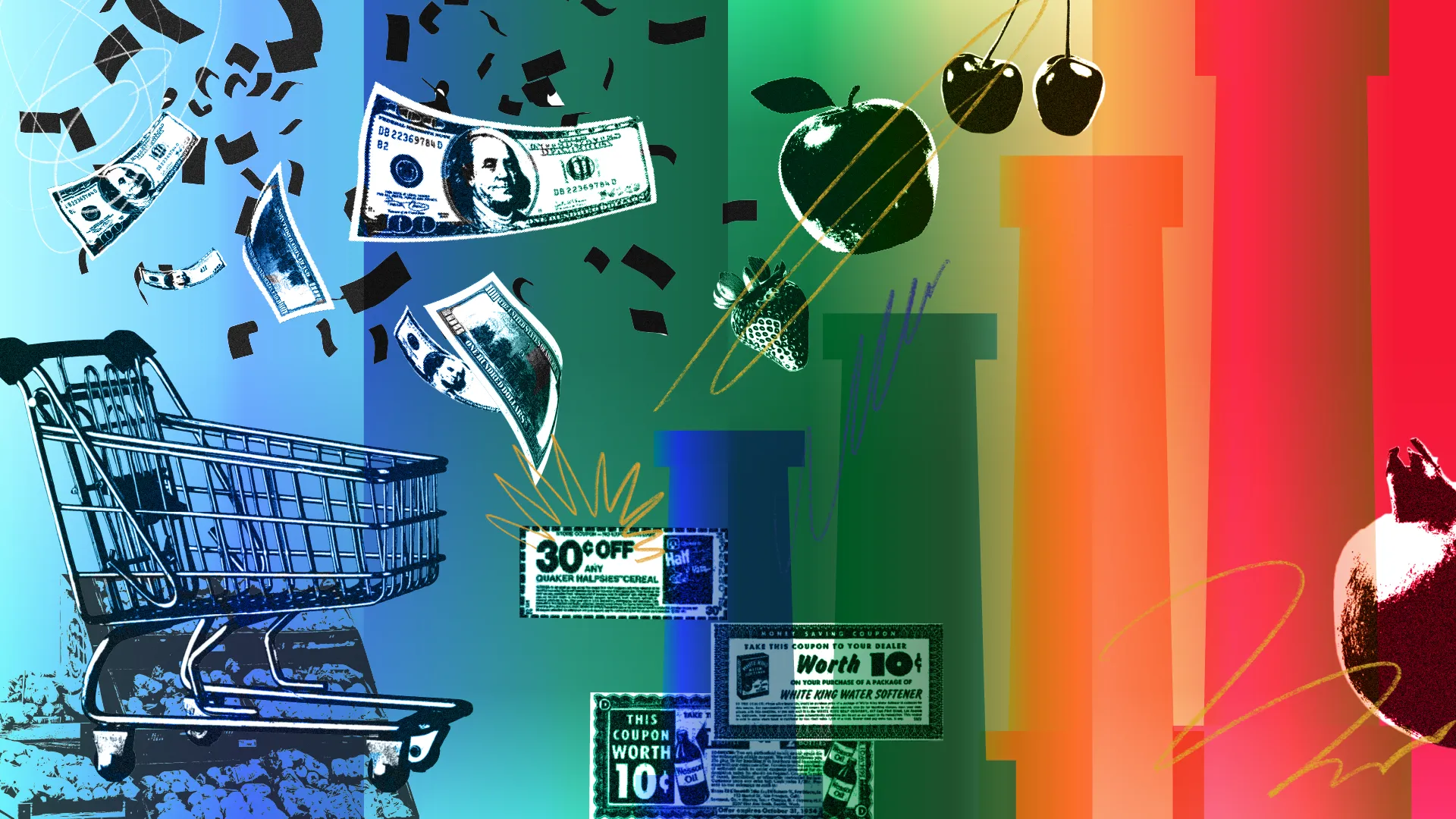
A/B Testing
Optimizing for Real Business Impact: A Strategic Framework for Ecommerce Experimentation
Learn more
If you’re like me, you’ve sat down in front of your A/B experimentation tool of choice and pressed F5 to refresh the page. And just like me, you didn’t just refresh once. You refreshed every ten minutes. Or five minutes. Or you refreshed after every page load because, why not, you can.
Unbeknownst to me at the time, there were downsides to the refresh addiction I had developed, which is better known in the A/B testing realm as “peeking”. And those downsides could easily have included totally incorrect decisions. There are alternative tools that I could have used that would soothe my anxiety while affording me the confidence my experiments were moving in the right direction.
As a product manager, running A/B tests is anxiety-inducing. PMs are goaled on making an impact, which means shipping features as often as possible to move your goal metrics. You spend time upfront in discovery and design to ensure you’re building the right solution to solve a user problem. Once you’ve released the feature, experimentation is the core tool you have to show leadership you’ve achieved your goals. That means all this effort and time leads you to experimentation software that shows you a different number for your performance every time you load it.
Every time I refreshed the A/B testing tool, I was trying to get that hit of dopamine. It’s like doomscrolling on social media and hoping the algorithm will serve me that divine piece of content that triggers the right part of my brain. But “doom-refreshing” an experiment comes with even greater consequences. I was hoping that on every next refresh, the number would go up and to the right. Because if it did, I wasn't just seeing a good piece of content, I was potentially meeting my quarterly goals, making the company more money, and putting myself in place for a bonus or promotion.
The problem is that looking at interim results too often can undermine the test through a phenomenon known as the "peeking problem". Peeking refers to checking the interim results of an A/B test before it has been completed. Most companies use a “fixed sample” test setup (a simple t-test being the most common), which assumes no one will peek at results during the experiment runtime.
The pitfalls of “peeking” at interim calculations in a fixed-sample test can be as dramatic as making the opposite decision of the true outcome. Statistical significance or p-value calculations aren’t making any allowance for your still-collecting sample size and may show something as a “stat-sig” win when it may be demonstrably harmful with enough data, or vice-versa. This is exacerbated by sophisticated tools offering updates in near-real-time, tempting us to refresh our dashboards with the hope of catching a positive trend.
But of course we likely want to get regular updates on how our ideas are performing in the real world. When an experiment is rolling out, we want to know that it’s configured correctly and that end users can use the experience we shipped. We also want to make sure we’re not causing any harm to site performance or key revenue metrics. Finally, we want to know if a primary metric is statically significant at an earlier point than expected, potentially allowing us to ship the experiment earlier, meaning we can make a greater impact sooner.
To account for this, modern A/B testing software often uses a sequential test. Sequential tests allow for peeking, but does so by requiring the sacrifice of some of the statistical power of the test, widening the final confidence intervals around our metrics. In other words, we’re making a trade-off of being able to peek at the results in return for longer running tests with less precise results. This tradeoff is correlated in magnitude too: the more peeks planned, the more power sacrificed. Knowing this trade-off, would we want to use Sequential tests or would we run Fixed Sample tests in certain circumstances?
Asking PMs to practice self-discipline and seek a deep understanding of statistics is not a solution. But fighting the urge to peek doesn't mean flying blind until the experiment concludes. Instead, there are strategic practices and tools that can offer peace of mind without compromising the integrity of experiments:
Incorporating these tools not only helps manage the peeking impulse but also ensures that our decisions are rooted in comprehensive, reliable data. By adopting a patient, methodical approach to experimentation, we can navigate the challenges of product development with confidence, ensuring that when we do look at our results, they're as meaningful and accurate as possible.
A/B testing with proper statistical methods is crucial for product development. But as I know all too well, impatience can undermine results. With the right mix of tools and knowledge of trade-offs, PMs can navigate the suspense of experiments with wisdom and confidence, ultimately leading to the results that lead to their career growth. If I can tackle my peeking problem, any PM can.