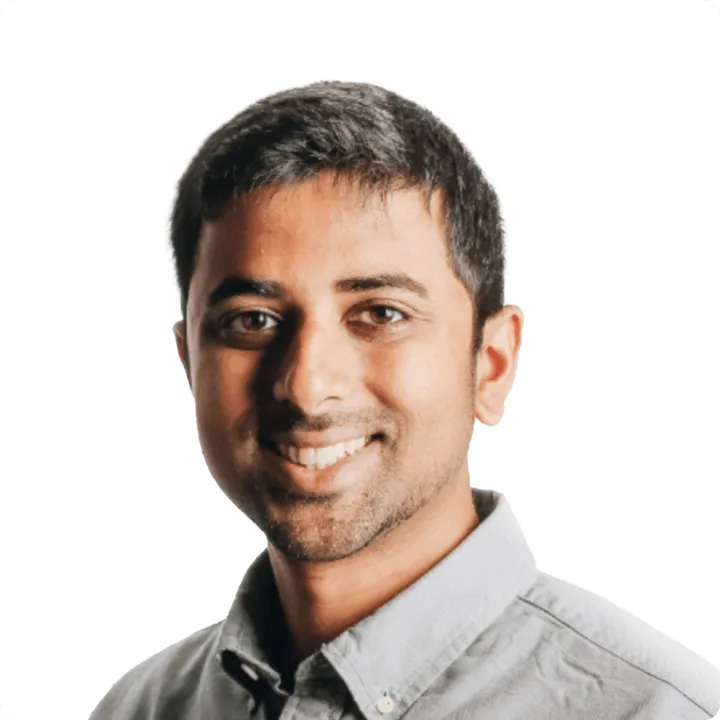
Che Sharma
August 12, 2021
Eppo's Founder and CEO, former early data scientist who built experimentation tools and cultures at Airbnb and Webflow
It's really a question of infrastructure and hypothesis generation
You often hear that great product-led-growth teams are running thousands of experiments a year, with the most sophisticated shops running every single product change as an experiment. Companies like Facebook, Airbnb, and Uber are able to do this strategy because they’ve invested in infrastructure that lowers experimentation overhead to zero.
But for most growth companies, these comprehensive experimentation strategies just aren’t practical. There’s just too much overhead per experiment to run experiments everywhere.
If experimentation at Airbnb is like turning on a stove, experimentation at most growth companies is like lighting a fire by rubbing sticks together. There’s a whole bunch of small things you wouldn’t do if you had to light fire this way, like birthday candles or making toast.
So when should you run an experiment? The best way to answer that question is to go through a two steps:
Let’s spell this out some more.
Experiments cost money. The money goes toward weeks of staff bandwidth, product development time, and maintenance of multiple code paths. If you trace through an experiment’s full lifecycle, there’s a lot of work involved:
If all of the above steps combined should take a few hours or less, congratulations! You can consider running experiments comprehensively, across every product launch. You also probably work at a handful of organizations like Uber, Airbnb, or Netflix, and have committed 10+ technical staff members to building infrastructure. You’re also likely so large that you can’t afford to have a product change go out that could make UX worse for a billion people, so you better be experimenting a lot.
For most growth companies' current infrastructure, the full lifecycle probably takes a collective month of people time. I’ve been at multiple companies where the monthly goal is to run a single experiment, recognizing that it’s hard to go through all of these hoops. Unfortunately, today's 3rd party tooling isn't helping lower experiment overhead. (This is what we hope to change at Eppo!) For these folks, you have to pick your spots.
A simple step that will drastically improve your experimentation practice is to make teams state their hypothesis, expected effect size, and development complexity on every experiment.
It shouldn’t come as a surprise that effect size and development complexity are key inputs to deciding on experiments. Both factors are important for product development in general. But the planning step that doesn’t always happen is making teams state a hypothesis. Saying a sentence like, “we believe that XXX will improve the customer experience by YYY leading to ZZZ” forces teams to clarify their justification for the experiment, and leads to organizational learning. For example:
🎉 Good Hypothesis: We believe that removing unnecessary information asks on the page will improve the customer experience by reducing friction leading to more purchases on the site.
🤢 Bad Hypothesis: We believe that swapping the location of this image and this paragraph will improve the customer experience by looking nicer leading to, ….uh well I’m not exactly sure.
To find great ideas, you might want to try answering the following before every learning experiment.
For example, before we had fully automated experimentation infrastructure, most of Airbnb's most impactful experiments came from ideas rooted in research, such as noticing that guests were sending messages to Airbnb hosts who rarely respond, or that hosts' have noticeable preferences in bookings they like. In a world where significant engineer, analyst, and product time overhead was involved per experiment, it was important to work on problems like these vs. button widths or full modal vs. half screen modals.
So when should you run experiments? It depends on your experimentation overhead and likely impact. People who strongly push for mass adoption of experimentation are usually doing so from a vantage point of sophisticated infrastructure and seamless workflows. They’re also likely working at a small number of decaunicorns who by necessity need the product hygiene from experimentation that prevents bad launches from reaching billions of people.
For most companies who are dealing with non-existent infrastructure and broken workflows, impact comes from choosing good hypothesis to test. Finding good hypotheses looks like normal product planning, yet many growth teams will run experiments with farcical justification that starts to look like throwing spaghetti at the wall. Skipping planning may seem like a way to increase speed to launch, but choosing good hypotheses leads to better speed to impact.